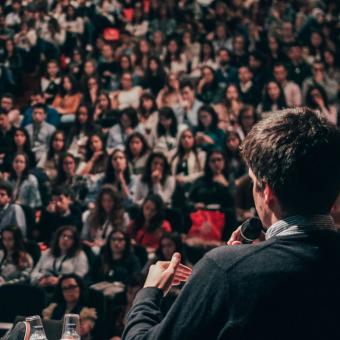
Psychiatry and psychology are based on assessing individuals' traits, relying vastly on behavioral testing and questionnaires. Imaging of brain activity raises the hope of measuring the biological differences that underlie these psychological variations. In the long run, imaging could not only reveal mechanisms, but also help refine the definition of relevant traits and diseases. However, imaging-based research faces multiple challenges: How to measure function in pathologies? How to relate experiments? Can understanding arise from data?
In this lecture, I will present one approach: massive data analysis on accumulation of rest fMRI across individuals. Rest fMRI is a good candidate for a universal marker of brain function, as it can easily be acquired on many different individuals. It reveals brain functional connectivity via "connectomes". The challenge is then to relate it to behavior and pathology. For this, we have developed a predictive-modeling pipeline; it successively defines regions from rest fMRI, build connectomes on these, and compares them across subjects using machine learning. My lecture will give the central ideas of such predictive connectomics, but also explore the new methodologies it opens for psychology and psychiatry.
Machine learning extracts from the connectomes the specific information relevant to predict a given clinical diagnosis or psychological score. However, it requires large cohorts which may lead to heterogeneity with uncontrolled confounds. Studying a multi-site autism cohort, we have shown that aggregating across site was beneficial even for a ill-define spectrum disorder such as autism.
Rest-fMRI may be combined with other modalities, that capture complementary information. For instance, brain aging induces specific changes in cortical thickness. I will show how such information can be merged in a predictive modeling approach to functional connectivity and lead to more accurate and more robust prediction.
Individuals are characterized by multiple clinical and behavioral information, including age, diagnostic status, a variety of psychological assessments. I will show how predicting these jointly leads to a better description of the individuals, including an improvement in diagnostic accuracy.
I will conclude with reflections on how these methodological developments, and those yet to come, can help shaping neuroimaging-based psychology and psychiatry. They face the challenge of the need for large cohorts, difficult to acquire and to control. Hence, they are complementary to focused study. Articulating wisely both approaches is a promising road to brain mechanisms.