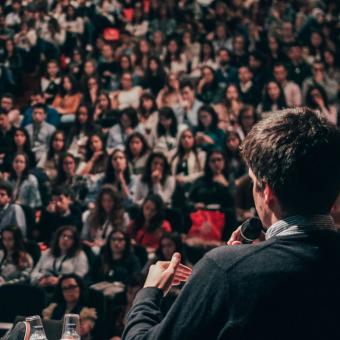
A network view of the brain has become ubiquitous in neuroscience. This has shifted focus from individual brain regions to macro-scale constructs including segregation and integration between remote regions. Complementing advances in multimodal non-invasive human imaging and signal processing, the mathematical tools that have allowed this perspective to flourish are graph theory and network science. By offering a constrained, yet expressive representation of large-scale, complex spatio-temporal data, graph approaches have enabled numerous systems-level insights into brain organization in health and disease. This talk will present mathematical tools that can be used to make sense of graph-structured brain data, both for group inference (graph-based statistics) and individual prediction (machine learning on brain graphs). We will particularly focus on applications in basic and clinical neuroscience, and show that graph-based approaches are particularly promising for inference across different levels and scales of brain biology.